Github link (most upto date roadmap / proposal here): https://github.com/yash98/journalist-bot
Knowledge Gathering: Utilizing minimal initial input to generate targeted questions that maximize information gathering by having a dialogue with people.
Knowledge Organization: Streamlining the structuring and analysis of gathered data for enhanced insights.
Another use case with a lot of overlap with the current one: Journalist bot - conduct interview with people to distill and preserve niche and novel information
Traditionally, surveys have predominantly consisted of multiple-choice and close-ended short answer questions. This is partly because analyzing responses to open-ended questions can be challenging. However, advancements in Natural Language Processing (NLP), such as Large Language Models (LLMs), have made it easier to tackle this issue. The efficiency and depth of traditional survey tools are often compromised by their inability to engage respondents fully, resulting in low participation rates and superficial data. The rigidity of preset questionnaires contributes to this problem, as they fail to adapt to the respondent’s unique perspectives or probe for deeper understanding. However, the evolution of machine learning, especially in natural language processing, now offers a solution to these challenges by enabling more nuanced interpretation of text-based responses.
Open-ended questions allow survey participants to express their thoughts and opinions more freely, potentially revealing insights that the survey creator hadn’t anticipated. In contrast, multiple-choice questions can inadvertently influence responses by presenting predefined options. However, open-ended questions also pose challenges, such as vagueness or lack of specificity, which may hinder the extraction of useful insights. Moreover, the process of identifying when a respondent’s answers lack clarity or context—and therefore require follow-up for clarification or additional detail—is traditionally manual, leading to inconsistency and missed opportunities for deeper data collection. In such cases, follow-up questions can help prompt participants to provide more specific information. Our focus is on addressing the challenges associated with open-ended questions, particularly regarding vagueness and staying aligned with the purpose of the question. This challenge is often only recognizable after the fact, underscoring the need for a more dynamic and responsive survey mechanism.
The introduction of a Large Language Model (LLM)-based survey tool revolutionizes data collection by dynamically interacting with respondents in a conversational manner. This tool is designed to understand and evaluate users’ responses in real-time, enabling it to ask follow-up questions that are tailored to the individual’s answers and the nuances within them. By employing a combination of advanced language understanding capabilities and real-time response evaluation, this application not only enhances engagement and participation rates but also ensures the collection of more detailed and meaningful data.

Presentation Link
- Setup backend, frontend, lm-server as mentioned in
README.md
in their respective folders
- Then UI can be access at
http://localhost:8501
- Recommended hardware Nvidia T4 GPU (you need about 16GB GPU RAM)
User opens our application - what do they see, what can they do there. With every user action what changes and are the the next possible user actions
- Create a new Survey
- Add topic
- Describe topic
- Add questions
- We will suggest some possible questions based on the topic and previously added questions
- Select question type -
- MCQ
- Text
- Creator specified configs -
- Maximum follow up question depth
- Question objective
- Criteria to evaluate whether to ask follow up questions or not
- Was answer specific?
- Was answer ambiguous?
- Was an example given in the answer?
- Did user understand the question? Did they answer the asked question or something else?
- Did user find the question irrelevant?
- Is question objective reached?
- With every question creator gets a field to explain / rephrase the question differently
- Suggest options using LLM
- Survey Analysis
- Research
- Can we use analysis to improve the follow up questions (P4)
Basic UI were user answers the configured questions one after the other



A fronted app written using streamlit can be used to create surveys and for filling survey
The fronted app interacts with a backend service written using FastAPI
The backend service contains the survey bot which use two agent - objective met agent, question generation agent to generate follow up questions wherever needed
The data for survey questions, conversation done with a survey participant and state of survey is stored in mongodb.
For LLM capabilities we host the model using vLLM which comes with a lot of LLM inference optimisations out of the box.
LLM used is quantised gemma-7b-it
We generated 20 surveys with questions (about 3 questions each survey) and associated motivation (some motivation were also added manually). We generated associated survey participant descriptions and question answers conversation based on survey questions. Then we sliced the conversations into multiple as the expected input by the agent and manually annotated the data (i.e. manually marked which conversation slice had which objectives met). This gave use approximately 100 test cases which we used to evaluate different prompts and thresholds for prompts
All the generations were done by prompt engineering and using GPT
Priority - P0 to P4
- Multiple type of questions
- MCQ (Single select and multi select) P1
- Text paragraph P0
- Multilingual Support P1
- Survey Bot (Collection of agents) P0
- Authentication P1
- Voice integration
- STT P3
- TTS P4
Yash Malviya, Santanu Senapati, and Pushkar Aggrawal, representing Search Relevance at Myntra, are honored to participate. With collective expertise, we aim to innovate solutions. Our team has worked on Gen AI enabled features and Deep Learning tools ex. MyFashionGPT for Myntra
Preserving and circulating knowledge across diverse domains can be challenging due to the sheer volume of information generated in various conversations. There is a need for a streamlined process to capture, distill, and present conversational knowledge in a readable format for broader consumption.
- Arnab (Journalist Bot): Arnab’s role is to facilitate discussions, ask relevant questions, and extract valuable knowledge from these conversations.
- Cataloging Pipeline: Converts Arnab’s recordings into a readable format, creating a dynamic encyclopedia.
- Consumption Platform: A user-friendly platform for exploring, searching, and validating knowledge across domains.
- Knowledge Preservation: Captures valuable insights, preventing loss.
- Knowledge Circulation: Breaks down domain barriers, encouraging cross-disciplinary learning.
- Collaborative Validation: Allows users to cross-reference information for accuracy and give feedback on the recorded information
- Continuous Learning: A growing encyclopaedia adapting to changing information, fostering continuous learning.
- The bot will interact with users in a non confrontational, curious and friendly manner. Asking relevant questions and keeping the conversation alive and easy. First POC is planned on OpenAI GPT interface however to enhance sticky conversation skills fine-tuning might be needed on data sources such as interviews and podcast transcripts.
- Bot should have multilingual support to enable wide variety of people to interact with bot and store their knowledge
- Distilling conversation into a transcript and writing unbiased digestible content such as a blog.
- Building a wikipedia-like repository of knowledge and keeping relevant data close by cataloging the generated blogs.
- If multiple instances of the same information are present it can be used to validate the information automatically. If differing opinions on the same topic is present we can detect that there is a chance of subjectivity in the topic
- The bot should ask questions that do not overwhelm. Do not ask a lot of questions to specific types of users, and it might be a bad experience for those users
- Some indigenous skills and cultural heritage such as Yoga, ayurveda, weaving, looming, handcraft techniques etc can be lost to time with advancement of technology, these can be preserved digitally with the help of this bot.
- Documentation of any tech product or scientific concepts.
- Journaling a trip.
- https://www.education.gov.in/nep/indian-knowledge-systems
- https://www.linkedin.com/pulse/preservation-promotion-indigenous-knowledge-21st-xholiso-ennocent/
- https://www.linkedin.com/pulse/role-indigenous-people-preserving-tradition-knowledge-glowsims/
Tasks to be solved -
- Translation
- If the language model is multilingual it’s not needed
- If not
- Bing translate API
- https://huggingface.co/spaces/facebook/seamless_m4t or https://huggingface.co/openai/whisper-large
- TTS
- https://huggingface.co/spaces/facebook/seamless_m4t or https://huggingface.co/openai/whisper-large
- STT
- https://huggingface.co/spaces/facebook/seamless_m4t or https://huggingface.co/openai/whisper-large
- Language Model
- Microsoft ChatGPT Open AI API
- We could Fine tuning LLM to make the conversation more engaging and to make smaller models more accurate for question generation task
- Data sets
- Podcast datasets (To make the conversation more engaging)
- https://zenodo.org/records/7121457#.Y0bzKezMKdY
- https://podcastsdataset.byspotify.com/#:~:text=The dataset contained over 100%2C000,take requests to access it. (No longer available)
- We can reuse QnA datasets, instead of generating answers we generate questions. If we have different QnA on a single topic, merging a list of question and answers and expecting the bot to generate the next question is our task
- SQUAD dataset is QnA over wikipedia article. We have the topic mentioned in title field https://huggingface.co/datasets/squad/viewer/plain_text/train?p=875
- Clustering on QnA Dataset to group similar QnA topics together
- Models
- Mixtral
- Llama
- https://huggingface.co/models?pipeline_tag=text-generation&sort=trending
- https://huggingface.co/models?pipeline_tag=question-answering&sort=trending
- Vector Search (Context for RAG)
- Dataset for retrieval
- https://python.langchain.com/docs/integrations/tools/google_search and other search tools
- Models
- https://huggingface.co/models?pipeline_tag=sentence-similarity&sort=trending
- Moderation
- https://python.langchain.com/docs/guides/safety/moderation
- Basic word based blacklisting / tagging
- Toxic
- Dangerous
- Bias
- Subjectivity
- Summarisation
- https://huggingface.co/models?pipeline_tag=summarization&sort=trending
- Text summarisation with Annotation
- Bot
- Stream Lit for UI
- Langchain and various other libraries for hosting models
- Cataloging pipeline
- Simple python script based indexing pipeline
- Periodic crons to generate summary of conversation topics
- Platform
- React for UI
- FastAPI for backend
- Databases
- Elastic search for search index database
- Mongo for document store
- Or depending on time left, in-memory databases
Evaluating our prompt (basically generated questions)
User Feedback based : https://medium.com/discovery-at-nesta/how-to-evaluate-large-language-model-chatbots-experimenting-with-streamlit-and-prodigy-c82db9f7f8d9
Generating questions in different contexts like -
- Artistic
- Political
- Technical
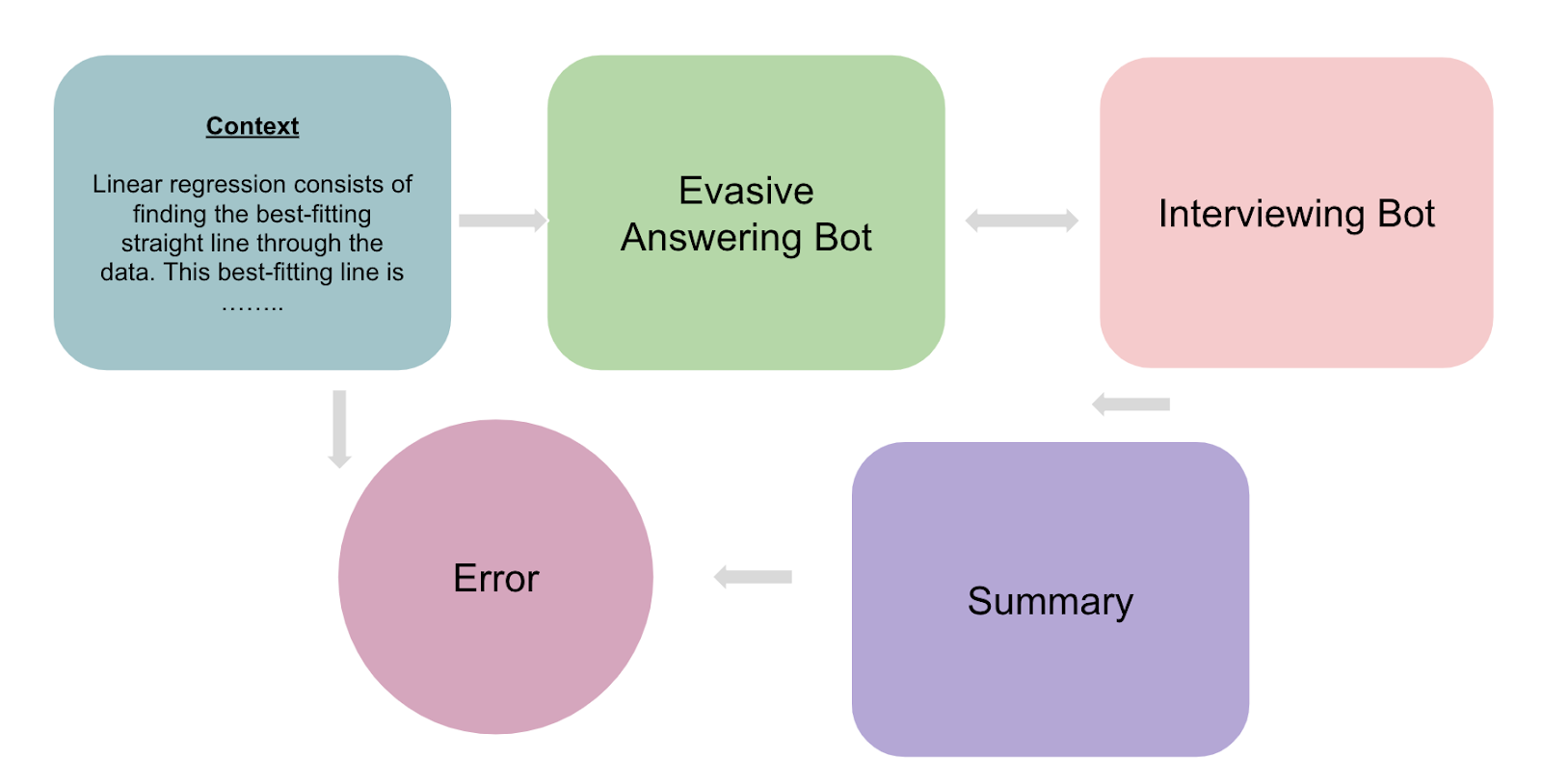
Task : Ask better questions
- Answering agent here becomes the examiner / evaluator
- Answering agent is provided a detailed context and exposed to the questioning agent. Answering agent is programmed to be vague and evasive.
- The questioning agent is exposed to the answering agent and at the end of their interaction we match the input context with the final questioning bot’s summary. SQuaD qualifies here as a prospect benchmarking dataset.
Answering agent can have different personalities like Easy going, difficult to talk to etc
- Chatbot - user interface
- Streamlit app for it’s simplicity and as team has familiarity with it
- Bot can start like - What do you want to document today
- User describes the topic to some extent
- Two options (We will decide exact one with POC)
- Generating questions
- Single prompt with instructions to be curious and friendly
- Or sequence of agents - Curious agent chained to friendly agent
- Fetch other relevant context (to evaluate)
- Unless the bot is somewhat aware of the content and challenges involved it might not be able to ask good questions
- User feedback - repeated question, irrelevant question (Streamlit has integration to take feedback too)
- Repeat questioning
- When to stop (POC needs to be done)
- Explicit action in the UI for the user to end the conversation
- Stopping implied by the conversation
- Giving the user an option to pick the conversation back up at any time will be useful
- Stores the conversation in a structured format automatically in the background
- Cataloging
- Moderation flagging
- Tagging the content for unsafe content to filter out for showing to others
- Conversation topic summarisation
- Showcase portal (will make a basic website)
- User experience (Like stack overflow + wikipedia)
- Search
- Moderation filtering
- View the conversation web page
- Comment / feedback section
- Topic summary page (collates different conversation from conversation pages)
- Comment / feedback section
- Traceback information to conversation it came from
- Component
- Moderation
- Subjectivity detection
- Bias detection
- Noting human crowdsourced validation of recorded information
- Chatbot
- Prompting POC
- Basic Streamlit UI
- TTS and STT integration
- Prompting engineering experiments
- Store conversation information in the background
- User feedback UI
- Cataloging
- Convert from format saved by bot to write in database
- Moderation tagging
- Conversation topic summarisation
- Platform
- Frontend functionality
- Search
- Basic Search page
- Moderation filtering
- View the conversation web page
- Basic conversation web page
- Comment / feedback section
- Topic summary page (collates different conversation from conversation pages)
- Basic Topic summary page
- Comment / feedback section
- Traceback information to conversation it came from
- Backend functionality
- Search build the index
- Search API
- Search Moderation filtering functionality
- Conversation web page view API
- Add Comment / feedback Conversation web page API
- Topic summary page view API
- Add Comment / feedback Topic summary page section
- Show traceback information to conversation it came from
{{ gettext('Login to leave a comment') }}
{{ gettext('Post a comment…') }}{{ errorMsg }}
{{ gettext('No comments posted yet') }}